Gemini Flash & Gemini 2.0: Revolutionizing AI Document Processing with Unmatched Cost Efficiency
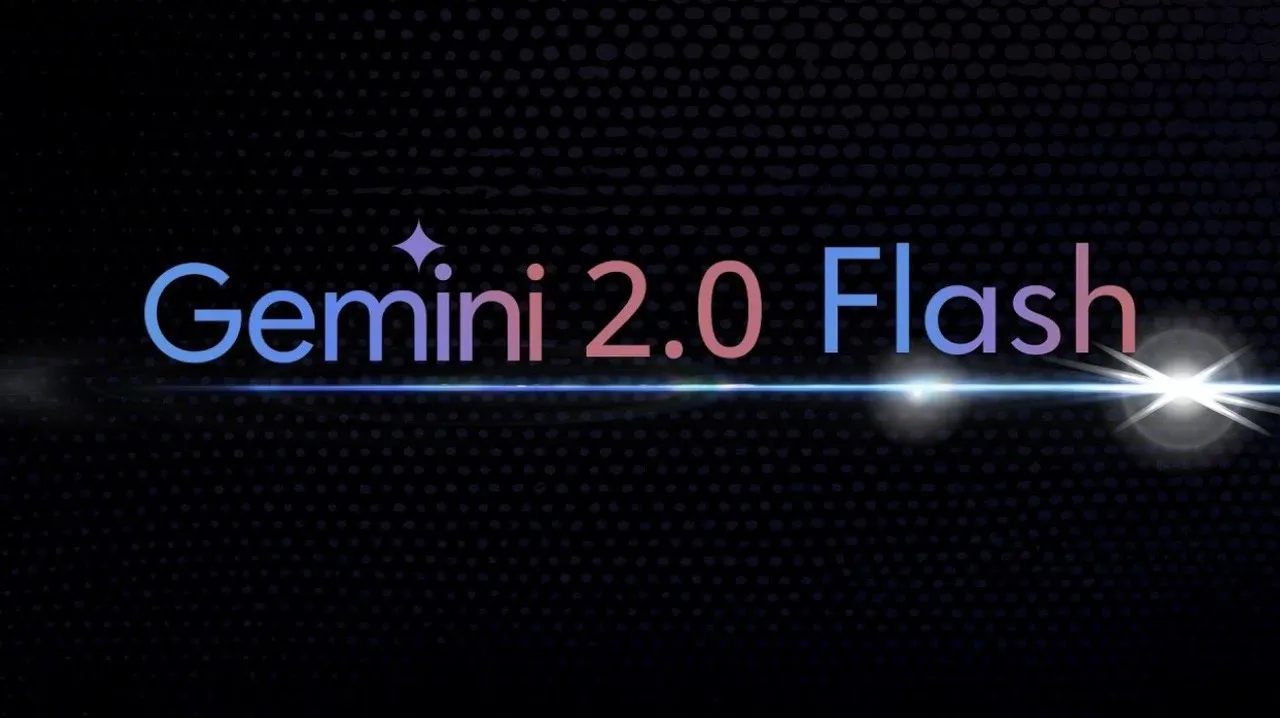
Build your custom chatbot with BotGPT
You can build your customer support chatbot in a matter of minutes.
Get StartedGemini Flash & Gemini 2.0: Revolutionizing AI Document Processing with Unmatched Cost Efficiency
Introduction
In today’s data-driven landscape, rapid and efficient document processing isn’t just a luxury—it’s a competitive necessity. Google’s Gemini family has recently taken center stage, offering an integrated solution that combines advanced OCR, layout comprehension, and intelligent chunking into one powerful model. On one hand, Gemini Flash has emerged as the “AI locksmith,” unlocking hidden insights from complex PDFs with incredible speed and cost efficiency. On the other, Gemini 2.0 pushes the boundaries further by leveraging multimodal inputs and an expansive context window to redefine how we ingest and analyze large volumes of documents.
Imagine processing thousands of PDF pages per dollar—an innovation that not only challenges legacy OCR vendors but also democratizes access to advanced AI technology for academia, legal research, business intelligence, and beyond. In this article, we explore both Gemini Flash and Gemini 2.0, breaking down their technical breakthroughs, cost efficiency, and the lively community debate surrounding them. We also examine their profound impact on document processing workflows and the economic implications for industries worldwide.
What Are Gemini Flash and Gemini 2.0?
Gemini Flash: The AI Locksmith
Gemini Flash represents a revolutionary approach to document processing. It’s engineered not only to extract plain text from dense documents but also to understand structured data like tables, charts, graphs, and diagrams. Traditional OCR tools merely transcribe text; Gemini Flash goes further by comprehending context and relationships within the data. This “AI locksmith” can sift through thousands of pages and deliver key insights in an accessible format.
Key features include:
- Advanced Document Comprehension: Through deep learning, Gemini Flash interprets structured elements—tables, charts, and images—providing an enriched understanding that once required human expertise.
- Unmatched Cost Efficiency: The tool can process up to 6,000 PDF pages per dollar in its standard configuration, with an even more impressive 12,000 pages per dollar in its Lite version. In stark contrast, industry competitors like OpenAI’s GPT-4 and Anthropic’s Claude process only 200 and 100 pages per dollar respectively.
- Rapid Processing Speed: Despite its low cost, Gemini Flash delivers near-instantaneous processing, making it ideal for time-sensitive projects and large-scale document ingestion.
Gemini 2.0: The Next Generation of Integrated Document Processing
Building on the successes of Gemini Flash, Gemini 2.0 expands the horizon with a fully integrated multimodal approach. It accepts not only text but also images and audio as inputs—making it exceptionally versatile for processing PDFs that blend scanned images with digital text. Key enhancements include:
- Multimodal Input Capabilities: Gemini 2.0 seamlessly processes diverse inputs, ensuring robust extraction even from mixed-content documents.
- Integrated OCR & Chunking: Rather than relying on separate tools for OCR, layout analysis, and text chunking, Gemini 2.0 performs all these tasks in a single pass. It converts complex documents directly into Markdown-ready text, efficiently splitting content into manageable chunks for downstream applications like retrieval-augmented generation (RAG).
- Enhanced Context Window: With support for context windows of up to 1 million tokens—and experimental versions reaching 2 million tokens—Gemini 2.0 can process entire documents or multi-page inputs without losing semantic structure.
- Cost Efficiency: Early benchmarks suggest that Gemini 2.0 can achieve similar cost savings to Gemini Flash. Although some analyses report conservative estimates of around 2,000 pages per dollar, optimal configurations claim up to 6,000 pages per dollar.
Technical Breakthroughs and Integrated Workflows
Traditional Versus Integrated Processing
Historically, document processing workflows have required multiple, often fragmented, steps:
- Image Conversion: PDF pages are converted into images.
- OCR Extraction: Dedicated OCR models then transcribe text from these images.
- Layout Analysis: Separate algorithms detect tables, charts, and document structures.
- Chunking & Formatting: Finally, extracted text is split into manageable segments (often in Markdown format) for further use.
Gemini 2.0 consolidates these steps into a single streamlined process. By accepting a PDF page as a multimodal input, it performs OCR, layout comprehension, and intelligent chunking in one go. This integration minimizes the need for orchestration between disparate services, thereby reducing technical complexity and lowering overall costs.
Token-Based Pricing: The Numbers Behind the Savings
Like many modern AI systems, Gemini models use a token-based pricing mechanism. Tokens are fragments of text (or encoded image data) used both as inputs and outputs. For instance, if a typical PDF page requires 500 tokens for full transcription, the cost efficiency (expressed as pages per dollar) is derived from these token calculations. Gemini’s optimized token usage allows it to dramatically undercut competitors:
Model | Pages per Dollar | Notes |
---|---|---|
Gemini 2.0 Flash (optimistic) | 6,000 | Integrated OCR & chunking; ideal conditions |
Gemini 2.0 Flash (conservative) | ~2,000 | Varies based on token usage and prompt tuning |
Gemini 1.5 Flash | 10,000 | Previous generation; less advanced but fast |
Amazon Textract | 1,000 | Traditional OCR vendor |
OpenAI GPT-4o | 200 | High-quality output at significantly higher cost |
Anthropic Claude 3.5 Sonnet | 100 | Lower throughput; expensive |
These figures illustrate a dramatic reduction in cost—a 30-fold improvement over GPT-4o and a 60-fold reduction compared to some legacy solutions. In practice, fine-tuning prompts to reduce unnecessary token usage can further push the boundaries of efficiency.
Comparative Analysis: Performance, Depth, and Economic Impact
Processing Speed and Depth of Analysis
Both Gemini Flash and Gemini 2.0 deliver on the promise of rapid data extraction without sacrificing depth of analysis. They are engineered for real-time processing, making them indispensable for tasks that require immediate insights from large volumes of data. The deep learning algorithms behind these models ensure that they not only extract text but also interpret the relationships within structured data—transforming raw PDFs into actionable intelligence.
Economic Impact on Research and Industry
The implications of such cost efficiency extend far beyond simple processing:
- For Academia: Researchers can now process thousands of pages without prohibitive costs, enabling deeper and broader studies without being bottlenecked by data extraction expenses.
- For Businesses: Companies can reallocate resources previously tied up in expensive OCR pipelines toward innovation, strategic planning, and research & development.
- For Startups: Lower barriers to entry allow emerging companies to access state-of-the-art document processing tools without significant capital expenditure, democratizing the technology.
Scalability and Integration
As organizations begin to integrate Gemini 2.0 into their workflows, the potential for scalable solutions becomes apparent. Whether it’s legal document analysis, business intelligence, or healthcare research, the ability to process large document corpora efficiently means that even small teams can handle tasks that were once the realm of large enterprises.
Community Reactions: A Spectrum of Insights
The launch of Gemini 2.0 has sparked a lively debate among developers and industry experts. Here are some rephrased and anonymized highlights:
-
Developer Experience:
“In our fintech firm, switching to Gemini 2.0 was a no-brainer. Its ability to handle multimodal inputs eliminated the need for multiple tools. Even though we noticed minor errors in handwritten text, the overall speed and simplicity far outweighed these issues.”
– A developer from a large financial institution. -
Cost Efficiency and Scalability:
“After benchmarking our pipeline, the savings were undeniable. Even on conservative estimates, processing thousands of pages for a fraction of the cost transforms the economics of document processing. This isn’t just incremental—it’s revolutionary.”
– An anonymous enterprise user. -
OCR Accuracy and Layout Analysis:
“I was impressed by how Gemini 2.0 handled complex layouts like multi-column PDFs and intricate tables. Minor misreadings were present, but for purposes like semantic search and summarization, these were negligible. However, precise bounding box extraction still lags behind specialized tools.”
– A veteran in document processing. -
Future Prospects and Market Disruption:
“This release is a wake-up call for legacy OCR vendors. Gemini 2.0’s cost and performance advantages will force the entire industry to innovate or face obsolescence. Its potential to democratize advanced document processing is particularly exciting for startups.”
– A tech forum participant.
Overall, while some concerns remain (particularly around spatial accuracy for certain niche applications), the consensus is that Gemini 2.0’s integrated approach and cost benefits are set to reshape the document processing landscape.
Implications for Traditional OCR Vendors and Hybrid Solutions
Simplifying Complex Workflows
By merging OCR, layout analysis, and chunking into one unified process, Gemini 2.0 simplifies workflows that traditionally required multiple, disparate services. This not only reduces the potential for errors but also cuts down on the time and resources needed for orchestration.
Pressure on Legacy Systems
For industries that rely heavily on document processing—ranging from legal firms to academic institutions—the economic benefits of Gemini 2.0 are compelling. As organizations switch to this unified model, legacy vendors may be forced to either lower their prices or innovate to remain competitive.
Hybrid Approaches for Precision Tasks
Despite its many advantages, Gemini 2.0 isn’t perfect. Tasks requiring pinpoint accuracy in bounding box extraction may still benefit from hybrid approaches that combine Gemini’s integrated processing with specialized OCR or object detection tools. Nonetheless, even these hybrid systems stand to gain from the underlying efficiencies of Gemini’s architecture.
Future Directions and Roadmap
Google’s commitment to refining the Gemini family is evident in ongoing updates and experimental models. Future improvements are likely to focus on:
- Enhanced Spatial Accuracy: Further refinements in bounding box detection and layout mapping to ensure that the digital representation of documents matches the source precisely.
- Expanded Multimodal Capabilities: Additional support for modalities such as video and higher-resolution image outputs will make the system even more versatile.
- Deeper Integration with Enterprise Workflows: Embedding these capabilities into platforms like Google Workspace will lower technical barriers for organizations and drive broader adoption.
- Hybrid and Customizable Solutions: Allowing developers to fine-tune the balance between cost, speed, and precision for niche applications will further extend the model’s utility.
Conclusion
Gemini Flash and Gemini 2.0 together mark a transformative moment in AI-powered document processing. By integrating advanced OCR, layout understanding, and intelligent chunking into a single, cost-efficient solution, Google has not only simplified complex workflows but also dramatically lowered the barrier to accessing high-end AI technology.
For researchers, business leaders, and developers alike, this unified approach offers unparalleled speed, depth, and scalability—empowering users to extract actionable insights from vast document libraries without prohibitive costs. While there are still areas for improvement, particularly in spatial precision, the overall impact is clear: Gemini 2.0 is set to redefine the future of document ingestion, driving down costs and catalyzing innovation across industries.
Embrace this new era of multimodal AI and discover how Gemini can unlock the hidden treasures within your data—one page at a time.